A new image enhancement method developed by Intel’s Intelligent Systems Lab allows computer-generated imagery to be enhanced with photorealistic graphics. Using GTA V as a demonstration, the deep-learning approach uses machine learning to analyze frames generated by the game. New frames are then generated from the real data. The technique in its research state may not be fast enough for a real-time playing experience, but it could be an important step forward for real-time computer graphics in the future.
Grand Theft Auto V’s San Andreas closely resembles LA and Southern California in real life, but this new project from Intel Labs called “Enhancing Photorealism Enhancement” might push it to be more photorealistic (via Gizmodo).
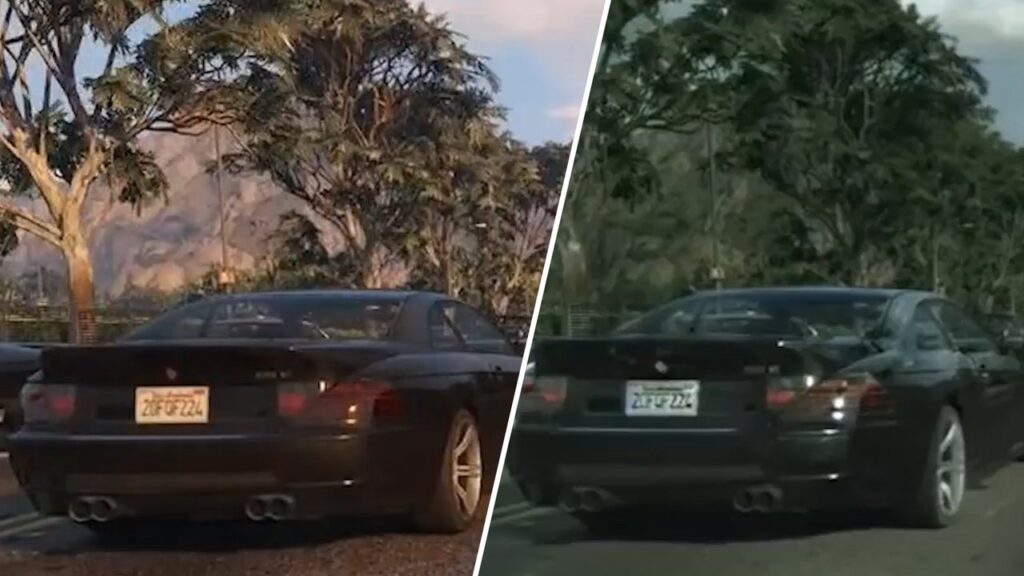
The game looks remarkably like the kinds of photos you might casually take through the smeared windows of your car when you run it through the processes Stephan R. Richter, Hassan Abu Alhaija, and Vladlen Kolten created. When seen in motion, it truly sells the idea that you’re looking at the real street from a real dashboard, even though it’s all virtual. The combination of slightly washed-out lighting, smoother pavement, and realistically reflective cars just sells it.
A portion of the photorealism can be attributed to the datasets Intel researchers fed their neural networks. As the group explains in greater detail in their paper (PDF), the Cityscapes Dataset used to build the enhanced images – built up largely from photographs of German streets – laid out much of the detail. Despite the dim light and the different view, it almost resembles Google Map’s Street View in a more interactive way. Despite not entirely acting like it’s genuine, it looks like it was made from genuine materials.
Additionally, the researchers added geometric information from GTA V itself to their enhancements, making them more accurate than standard photorealistic conversion processes. These “G-buffers,” as the researchers describe them, can contain information such as the distance between objects in a game and the camera, or the quality of texture information such as how shiny a car is.
GTA V won’t get a “photorealism update” tomorrow. But you’ve probably already played a game or viewed a video that made use of an AI algorithm — upscaling. The process of boosting graphics with machine learning doesn’t appear everywhere, but is featured on Nvidia’s Shield TV as well as several mods designed to upgrade the graphics of older games. These are instances when a neural network is used to override lower resolution details in a game, film, or TV show to reach the higher resolutions needed for those games, movies, and shows.
It’s probably not the only goal for graphics in video games (artistically, it looks creepy), but Intel Labs’ work illustrates that software could make as much progress in the coming years as raw GPU power does.
Image enhancement using deep learning
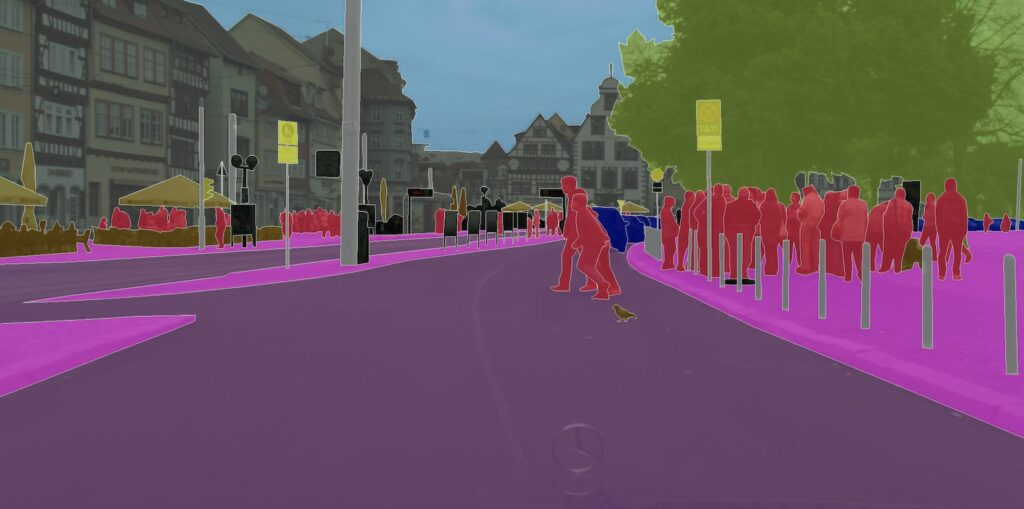
Deep learning systems developed by Intel have not been described in detail. The authors of the paper and video have released a video on YouTube that provide valuable insight into how much computation power you’ll need to run this model.
By encoding different render maps (G-buffers) into numerical features, the G-buffer encoder transforms them. G-buffers contain information on surface normals, depth, albedo, glossiness, atmosphere, and object segmentation. The neural network combines convolution layers to process this information and generates 128 features which enables it to better perform than other similar techniques and avoid unintended artifacts. Directly from the game engine, G-buffers are retrieved.
An image enhancement network is used to generate the photorealistic version based on the game’s rendered frame and the G-buffer encoder features.
Training uses the discriminator and loss function of the LPIPS algorithm. By examining the consistency of their output with the game-rendered frame and comparing its photorealistic quality with actual images, they grade the output of the enhancement network.
Estimating the cost of improving images
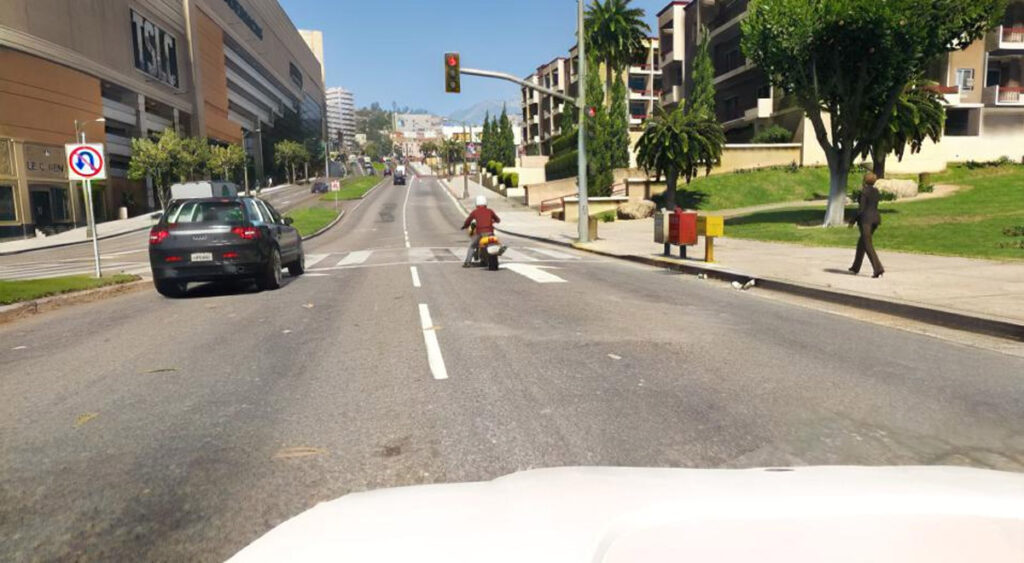
The bulk of the project is focused on the enhancement network. This neural network reportedly uses HRNetV2, an architecture used for deep learning that processes images of high resolution. The artifacts in neural networks that are capable of processing high-resolution images are fewer than those produced by downsampling.
Using multiple branches that operate at different resolutions, Intel’s paper explains that HRNet processes images. To preserve fine image structure, one of the feature streams is kept high resolution (1/4th of the input resolution).
For example, if you run the game at full HD (1920*1080), then the top layer will process input at 480×270 pixels. Each lower row has a halved resolution. Rather than merely computing inputs from the input encoder block (the RAD layers) in the neural network, the researchers changed the structure of each block to include G-buffer encoder inputs.
Conclusion
The gaming industry lacks engineers who specialize in machine learning, which adds to the cost of the task. There will be a decision to be made by the company about whether a photorealistic rendering makes the games more enjoyable.
In Intel’s photorealistic image enhancer, AI algorithms show just how far they can go. Real-time AI-based photorealistic rendering, however, is more than a few years away from becoming a reality.