A baby is more dexterous than a robot even at the age of one year. Sure, machines can do more than pick up and place objects, but we’re still a long way from mimicking a natural need for exploratory or dexterous manipulation.
OpenAI tried it out with “Dactyl,” a humanoid robot hand that solves a Rubik’s cube using software that’s a step toward more generic AI and away from the traditional single-task approach. “RGB-Stacking,” a vision-based system developed by DeepMind, tests a robot’s ability to grasp and stack objects.
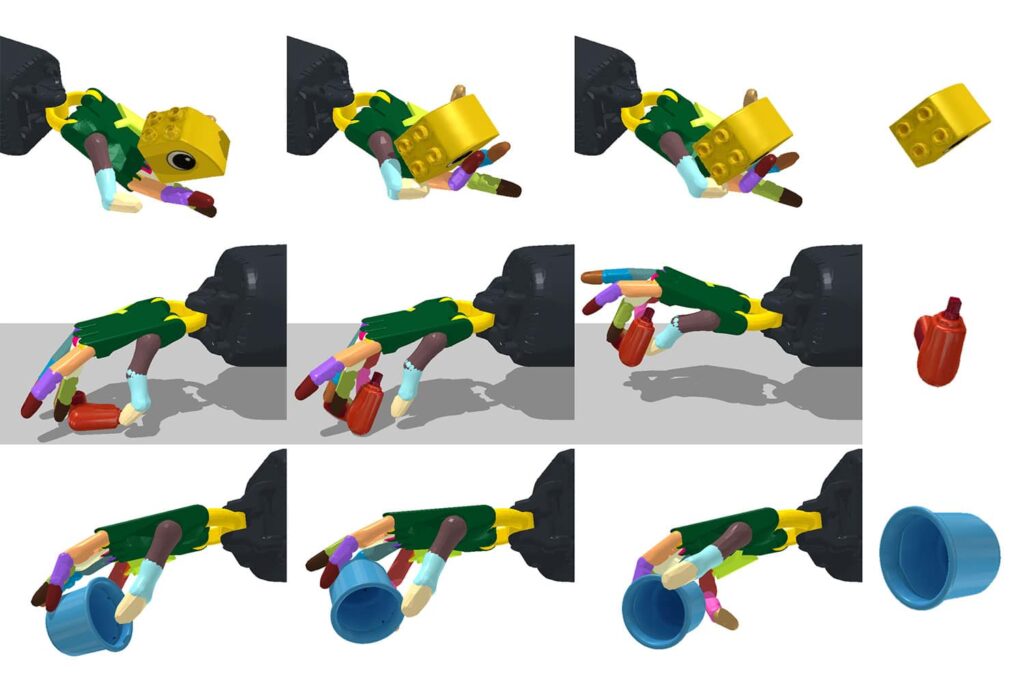
In the never-ending quest to get machines to mimic human abilities, scientists from MIT’s Computer Science and Artificial Intelligence Laboratory (CSAIL) developed a more scaled-up framework: a system that can reorient over two thousand different objects, with the robotic hand facing both upwards and downwards. This capacity to manage everything from a cup to a tuna can and a Cheez-It box could aid the hand in quickly picking and placing objects in specified ways and locations — and even generalise to objects that aren’t visible.
This dexterity “handiwork,” which is normally limited to single jobs and upright positions, could be useful in logistics and production, assisting with basic demands like putting goods into slots for kitting or managing a larger range of tools. The team demonstrated that the technology may be converted to a true robotic system in the future using a simulated anthropomorphic hand with 24 degrees of freedom.
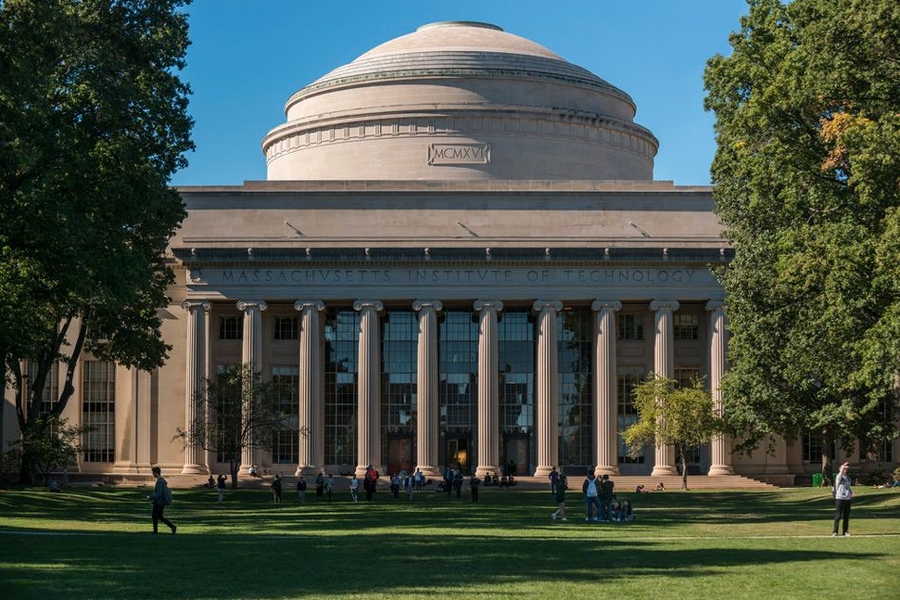
“In industry, a parallel-jaw gripper is most commonly used, partially due to its simplicity in control, but it’s physically unable to handle many tools we see in daily life,” explains Tao Chen, an MIT CSAIL PhD student and the project’s primary researcher. “Even using a plier is difficult because it can’t dexterously move one handle back and forth. Our system will allow a multi-fingered hand to dexterously manipulate such tools, which opens up a new area for robotics applications.”
Please lend us a hand.
Due to the huge number of motors to be controlled and the rapid change in contact state between the fingers and the objects, this form of “in-hand” object reorientation has been a difficult problem in robotics. The model had a lot to learn with almost two thousand objects.
When the hand is looking downwards, the task gets even more difficult. Not only does the robot have to control the thing, but it also has to avoid falling down.
The team discovered that a straightforward strategy may be used to solve complicated issues. They combined deep learning with a model-free reinforcement learning algorithm (which means the system has to figure out value functions from interactions with the environment) and a “teacher-student” training technique.
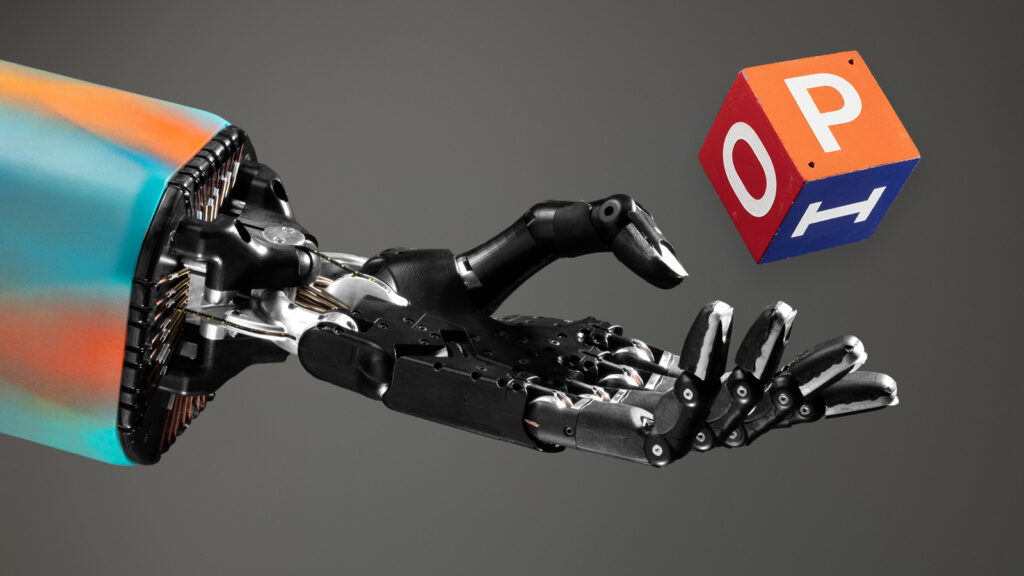
The”teacher”network is trained on information about the object and robot that is readily available in simulation but not in the actual world, such as the placement of fingertips or object velocity, enabling this to operate. To ensure that the robots can function outside of the simulation, the “teacher’s” knowledge is distilled into real-world observations, such as depth images obtained by cameras, object pose, and the robot’s joint positions. They also used a “gravity curriculum,” in which the robot initially learns the ability in a zero-gravity environment before gradually adapting the controller to normal gravity. Taking things at this pace significantly enhanced overall performance.
While it may seem contradictory, a single controller (referred to as the robot’s brain) was capable of reorienting a vast number of objects it had never seen before, and with no prior knowledge of shape.
“We initially thought that visual perception algorithms for inferring shape while the robot manipulates the object was going to be the primary challenge,” says MIT professor Pulkit Agrawal, who is also an author on the research paper. “On the contrary, our findings suggest that robust control techniques that are shape agnostic can be learned. “To the contrary, our results show that one can learn robust control strategies that are shape agnostic. This suggests that visual perception may be far less important for manipulation than what we are used to thinking, and simpler perceptual processing strategies might suffice.”
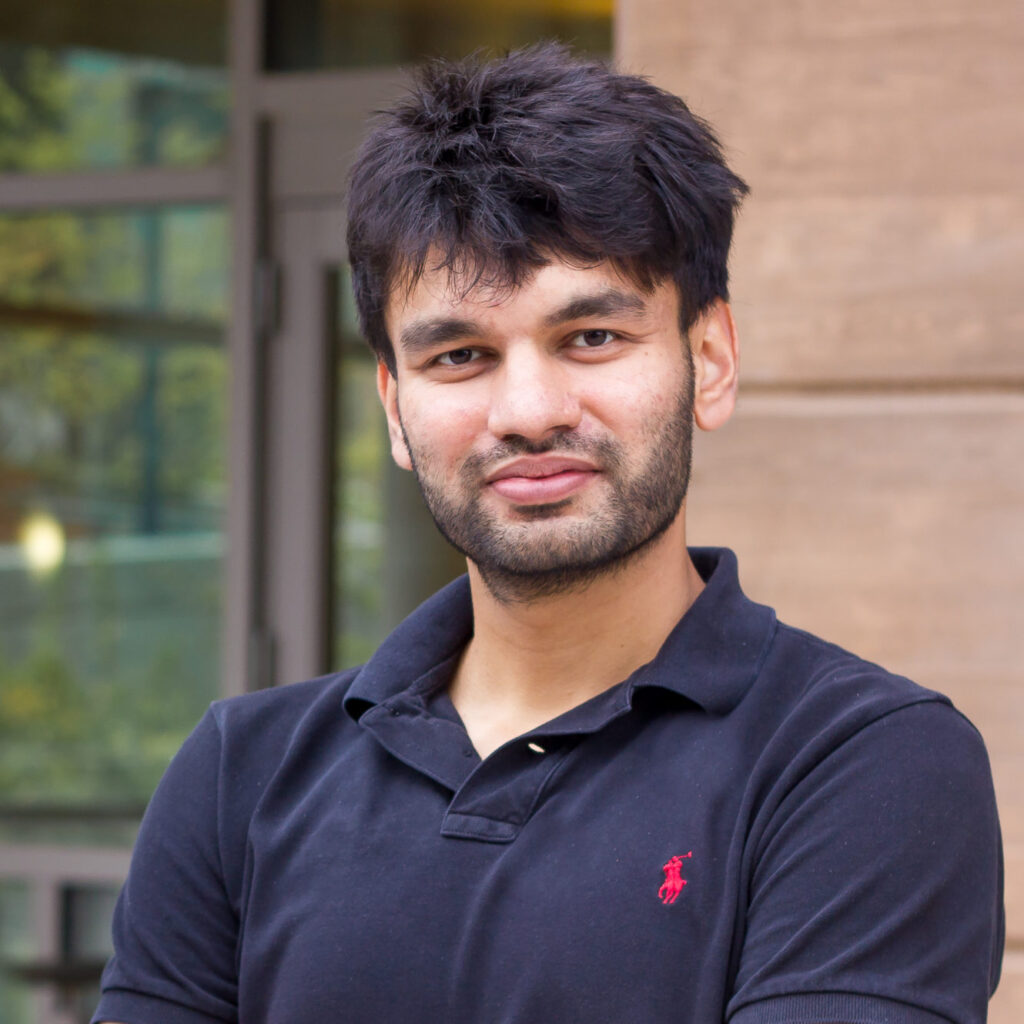
When reoriented with the hand facing up and down, many small, circular shaped objects (apples, tennis balls, marbles) had near-one hundred percent success rates, with the lowest success rates, unsurprisingly, for more complex objects like a spoon, screwdriver, or scissors, being closer to thirty percent.
Because success rates vary with object shape, the team believes that training the model based on object forms could increase performance in the future.
Chen co-authored a paper on the topic with Jie Xu, an MIT CSAIL PhD student, and MIT professor Pulkit Agrawal. Toyota Research Institute, Amazon Research Award, and DARPA Machine Common Sense Program financed the study. It will be presented at the Robot Learning Conference in 2021.