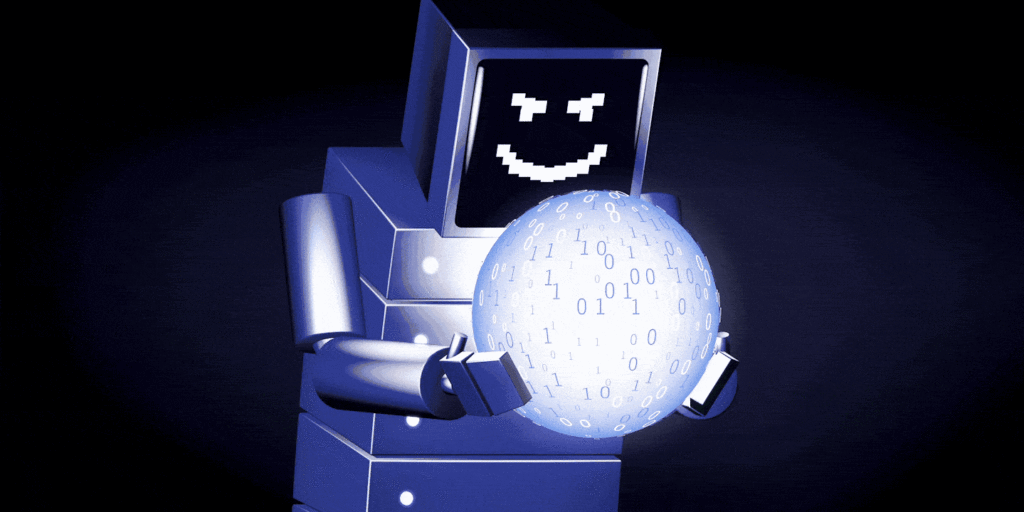
Facebook has introduced Anticipative Video Transformer (AVT), a new machine-learning method that uses visual interpretation to forecast future behaviours. AVT is an attention-based approach for action anticipation in videos that operates from beginning to conclusion.
The new model is built on recent developments in transformer topologies, notably for natural language processing and image modelling for self-driving vehicles and augmented reality applications.
AVT examines an action to determine the likely outcome, with a focus on AR and the metaverse. Through APIs that allow programmes to communicate with one another, Facebook intends for its metaverse apps to function across platforms and devices.
Future activity prediction is a tough problem for AI since it requires both forecasting the multimodal distribution of future activities and modelling the trajectory of existing actions.
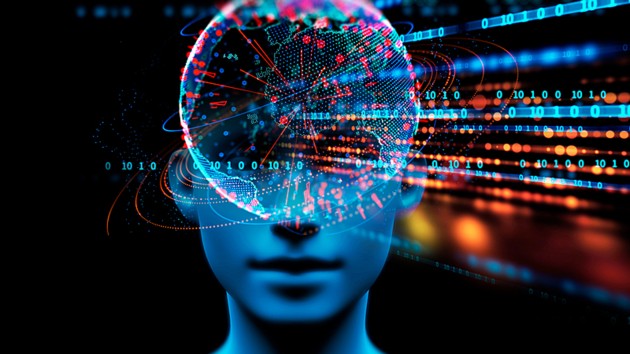
Because AVT is attention-based, it may analyse an entire sequence in parallel, whereas recurrent-neural-network-based techniques must process sequences sequentially and sometimes forget the past. Loss functions in AVT allow the model to capture the sequential character of video, which would otherwise be lost in attention-based designs like nonlocal networks.
AVT is made up of two parts: an attention-based backbone (AVT-b) that works with video frames and an attention-based head architecture (AVT-h) that works with the backbone’s features.
The vision transformer (VIT) architecture is used to build the AVT-b backbone. It divides frames into non-overlapping patches, uses a feedforward network to embed them, adds a particular categorization token, and applies many levels of multihead self-attention. The head architecture takes the per-frame characteristics and applies a causal attention transformer architecture. This implies it only considers characteristics from the current and previous frames. As a result, the model can generate a representation of every specific frame only based on previous characteristics.
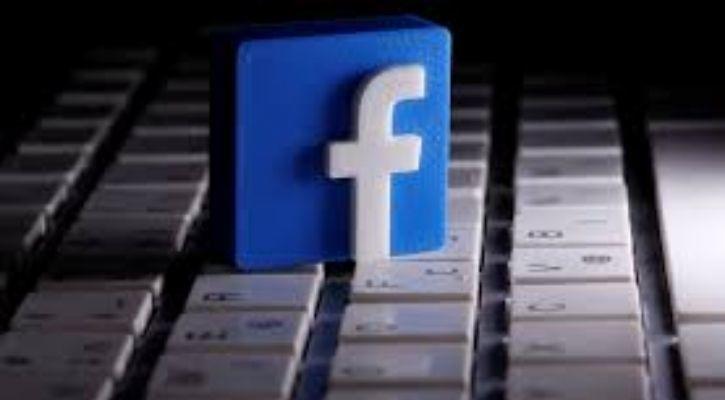
AVT might be utilised as an augmented reality action coach or as an AI assistant that warns individuals before they make mistakes. AVT might also be useful for tasks other than anticipation, such as self-supervised learning, discovering action schemas and bounds, and even general action recognition in tasks that involve modelling the temporal sequence of activities.