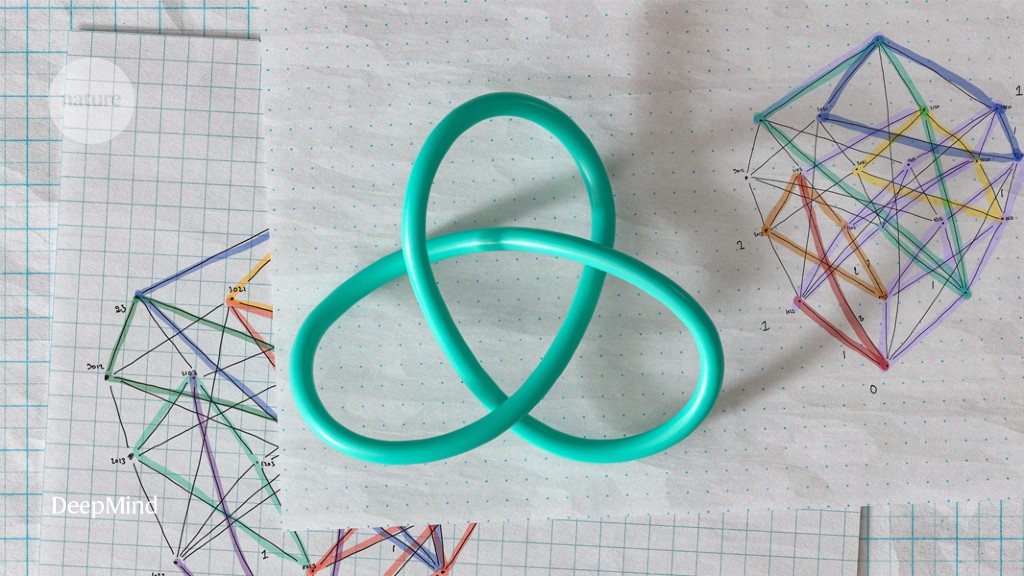
Machine learning has discovered mathematical relationships that humans have overlooked for the first time. DeepMind, a London-based artificial-intelligence powerhouse, collaborated with mathematicians to solve two unrelated challenges, one in knot theory and the other in the study of symmetry. AI tools aided the researchers in both situations in discovering new patterns that could subsequently be studied using traditional methods.
“I was very struck at just how useful the machine-learning tools could be as a guide for intuition,” says Marc Lackenby at the University of Oxford, UK, one of the mathematicians who took part in the study. “I was not expecting to have some of my preconceptions turned on their head.”
According to Jeffrey Weeks, a mathematician based in Canton, New York, who has pioneered some of those approaches since the 1980s, computer simulations and representations of knots and other objects have long helped mathematicians hunt for patterns and enhance their intuition. “Getting the computer to seek out patterns, on the other hand, takes the study process to a qualitatively other level,” he says.
The technique, reported in a study published in Nature1 on December 2nd, might aid other fields of mathematics that deal with enormous data sets, according to the authors.
Machine vs. mathematics
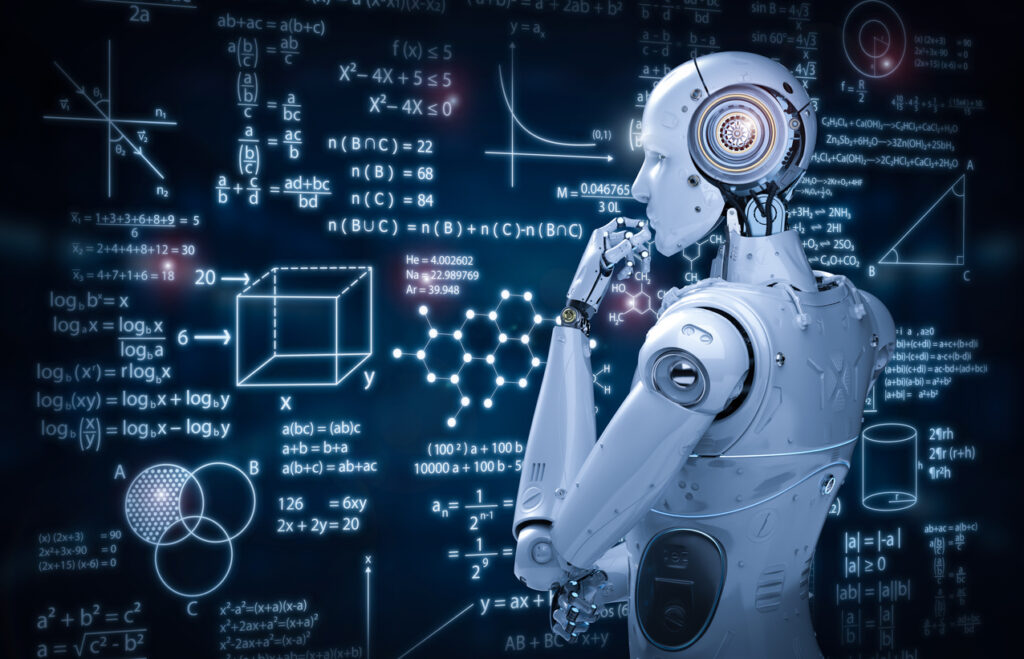
DeepMind, a Google subsidiary, has made headlines for triumphs like beating Go, but its long-term focus has been on scientific applications like predicting how proteins fold.
A casual encounter between mathematician Geordie Williamson of the University of Sydney in Australia and DeepMind’s chief executive, neurologist Demis Hassabis, in 2019 prompted the idea for a mathematical partnership. Lackenby and András Juhász, a colleague at Oxford and a knot theorist, were quick to join the project.
Initially, the effort centred on discovering mathematical problems that DeepMind’s technology may be utilised to solve. Machine learning allows computers to make educated assumptions based on big data sets, such as matching a security camera picture to a recognised face from a database of photos. However, its responses are probabilistic by nature, and mathematical proofs demand certainty.
Machine learning, on the other hand, might aid in the detection of patterns, such as the association between two sorts of objects, according to the study. Mathematicians might next try to figure out the exact link by creating a hypothesis and then writing a thorough proof that transforms that assertion into a certainty.
Because machine learning requires a significant amount of data to train on, one requirement was the ability to compute attributes for a large number of objects: in the case of knots, the team calculated various qualities, known as invariants, for millions of distinct knots.
The researchers next set about determining which AI method would be most useful in identifying a pattern that connected two attributes. One approach in particular, known as saliency maps, proved to be quite beneficial. It’s frequently used in computer vision to figure out which regions of a picture contain the most important data. Saliency maps identified knot qualities that were likely connected to one another, and a formula was constructed that appeared to be true in all situations that could be evaluated. Lackenby and Juhász then proved that the formula was correct for a large class of knots.
“The fact that the authors have proven that these invariants are related in such a direct way shows us that there is something fundamental that we in the field have yet to fully understand,” says Mark Brittenham, a knot theorist at the University of Nebraska–Lincoln who frequently uses computational techniques. Although machine learning has been employed in knot theory before, the authors’ method is unique in its capacity to uncover unexpected connections, according to Brittenham.
Identifying and resolving symmetries
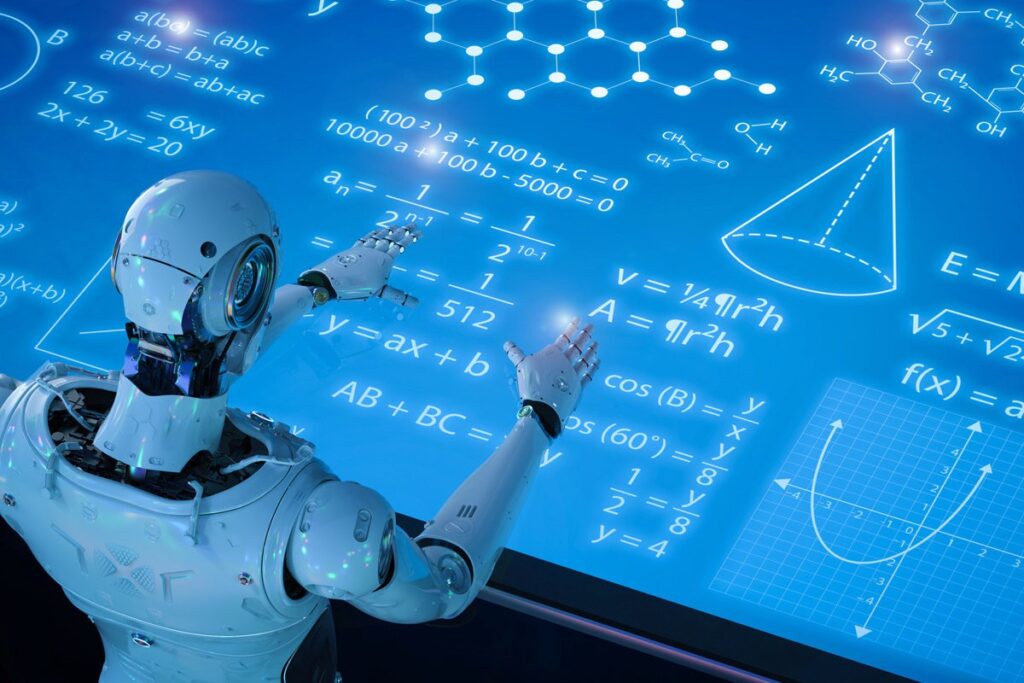
Williamson concentrated on a different issue involving symmetries. Mathematicians have long investigated symmetries that flip around finite sets of objects using a variety of methods, including graphs — huge abstract networks linking millions of nodes — and algebraic formulas called polynomials. Researchers have speculated for decades that the polynomials might be calculated from the networks, but figuring out how to do so looked hopeless, according to Williamson. “The graph rapidly becomes incomprehensible to humans.”
He and the rest of the team realised that the graph could be broken down into smaller, more manageable portions, one of which had the structure of a higher-dimensional cube, with the aid of the computer. For the first time, Williamson had a strong hypothesis to work with.
Williamson adds, “I was absolutely blown away by how strong this material is.” The programme was able to predict which graphs and polynomials stemmed from the same symmetries when it honed down on a pattern. “How rapidly the models gained accuracy — it was astounding to me,” he adds. “I’m quite sure I spent a year in the dark just hoping the computers knew something I didn’t.”
It’s still unclear if Williamson’s prediction will come true. Conjectures can take a long time to be debunked by the mathematical community, yet they can contribute to shape entire sciences.
Applications that are more diverse
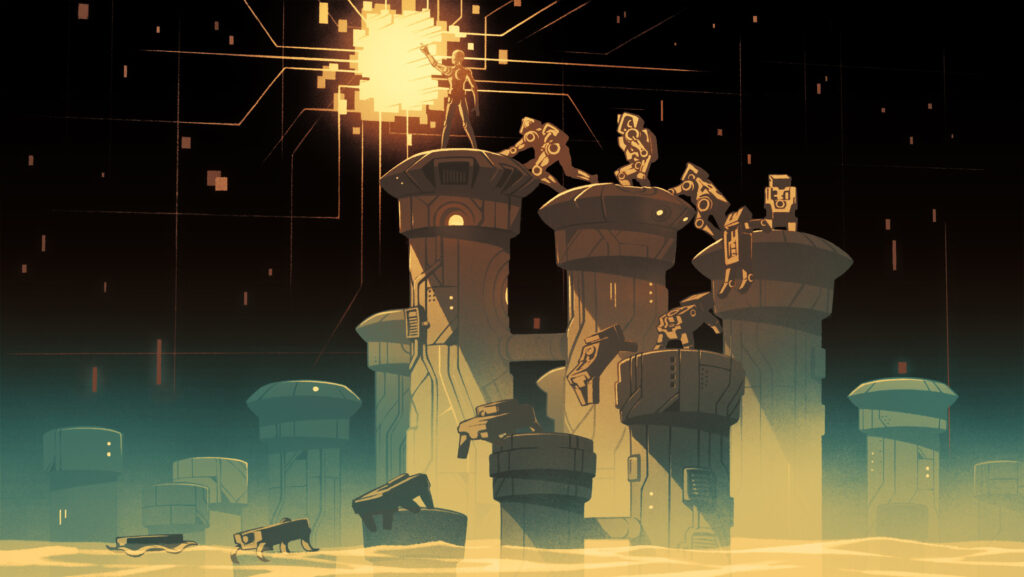
According to Alex Davies, a computer scientist at DeepMind, the researchers had to adjust the AI algorithms to the two separate mathematical issues throughout the project. “We didn’t think these would be the most beneficial tactics at first,” he explains.
“This strategy might assist any area of mathematics where sufficiently big data sets can be collected,” Juhász says, adding that the approaches they presented could potentially find applications in domains like biology or economics.
According to Adam Zsolt Wagner, a mathematician at Tel Aviv University in Israel who has worked with machine learning, the authors’ approaches might be useful for specific issues. “Without this instrument, a mathematician may squander weeks or months attempting to establish a formula or theorem that would eventually be shown erroneous.” However, he notes that the extent of its influence is unknown.
Davies told reporters at a news conference that the endeavour had given him a “genuine respect” for mathematical study. He went on to say that learning arithmetic in school is like to playing scales on a piano, but actual mathematicians’ work is more analogous to jazz improvisations.
Williamson acknowledges that the work reveals a side of mathematics that is less well-known. “We live in a world replete with intuition and imaginations as mathematical researchers,” he explains. “So far, computers have serviced the dry side. They are assisting with the other side, which is why I enjoy this work so much.”
“My personal guess is that computer-generated conjectures will become ever more useful in ‘filling in the details’, but will never replace human intuition and creativity,” says Weeks.