At least in the eyes of artificial intelligence, how you engage with a crowd can help you stand out.
Researchers report in Nature Communications on January 25 that when given information about a target individual’s mobile phone interactions as well as the interactions of their contacts, AI can correctly pick the target out of more than 40,000 anonymous mobile phone service subscribers more than half of the time. The findings imply that humans socialise in ways that could be used to identify them in apparently anonymised databases.
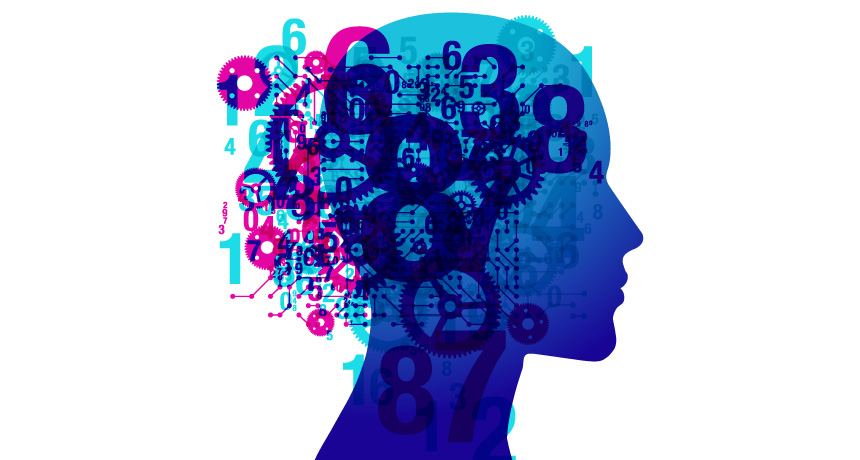
According to Jaideep Srivastava, a computer scientist at the University of Minnesota in Minneapolis who was not involved in the work, “it’s no surprise that people tend to stay within established social circles and that these frequent contacts develop a stable pattern over time.” “However, it’s surprising that you can utilise that pattern to identify the individual.”
Companies that collect information about people’s daily activities can share or sell this data without their consent, according to the General Data Protection Regulation of the European Union and the California Consumer Privacy Act. The only snag is that the information must be anonymised. According to Yves-Alexandre de Montjoye, a computational privacy expert at Imperial College London, some businesses may believe they can achieve this criteria by giving users pseudonyms. “Our findings suggest that this is not the case.”
People’s social conduct, de Montjoye and his colleagues argued, may be used to identify individuals in databases including information on anonymous users’ interactions. To test their hypothesis, the researchers trained an artificial neural network to spot patterns in users’ weekly social contacts. An artificial neural network is an AI that models the neural architecture of a biological brain.
The neural network was trained with data from an undisclosed mobile phone provider that tracked 43,606 subscribers’ interactions over a 14-week period for one test. The date, time, duration, type (call or text), pseudonyms of the people engaged, and who initiated the conversation were all included in this data.
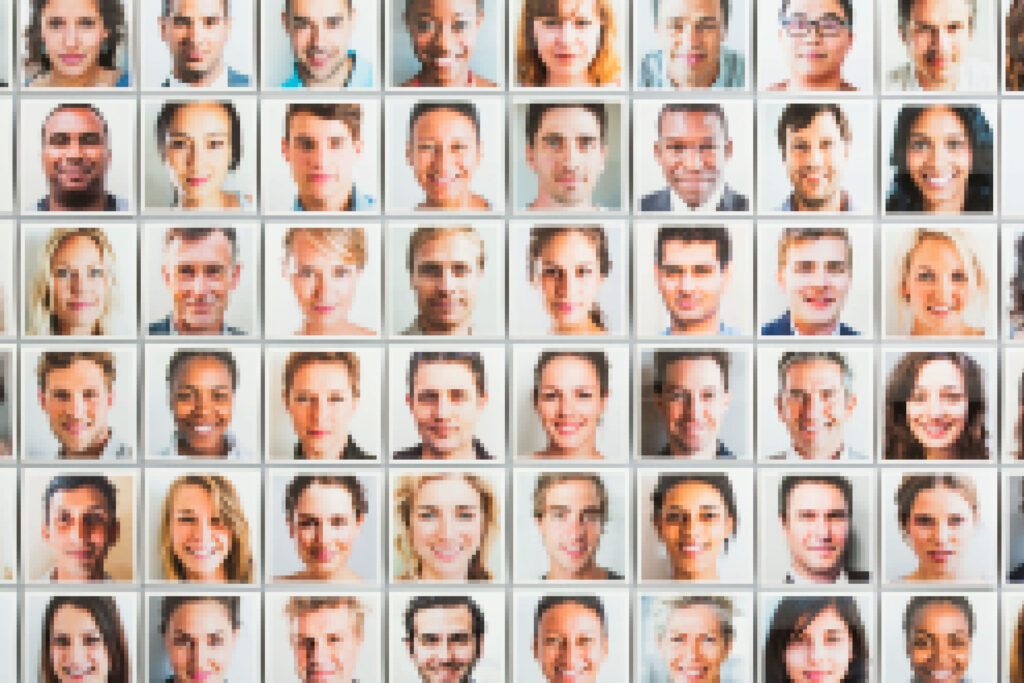
The interaction data of each user was arranged into web-shaped data structures, with nodes representing the person and their contacts. The nodes were joined by strings threaded with interaction data. The AI was presented a known person’s interaction web and then given free reign to search the anonymized data for the web that looked the most like it.
When provided interaction webs containing information about a target’s phone conversations that occurred one week after the latest records in the anonymous dataset, the neural network only connected 14.7 percent of individuals to their anonymised self. When provided information on the target’s interactions as well as those of their contacts, it was able to identify 52.4 percent of people. When the researchers fed the AI interaction data from the target and contacts 20 weeks after the anonymous dataset, the AI accurately identified users 24.3 percent of the time, implying that social conduct can be tracked for lengthy periods of time.
The researchers used a dataset of four weeks of close-proximity data from the mobile phones of 587 anonymous university students obtained by academics in Copenhagen to explore if the AI could analyse social behaviour elsewhere. This information contained pseudonyms for students, encounter times, and the strength of the received signal, which indicated closeness to other students. COVID-19 contact tracing software frequently collect these metrics. The AI properly recognised students in the sample 26.4 percent of the time when given a target and their contacts’ interaction data.
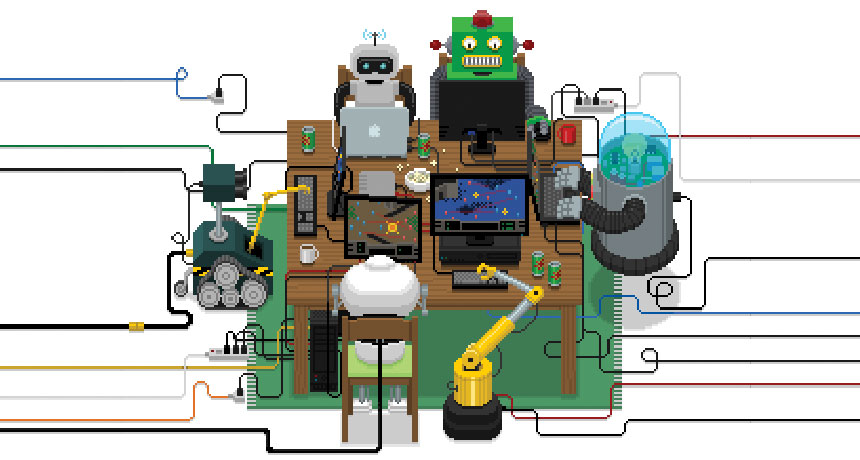
The findings, according to the researchers, are unlikely to apply to Google’s and Apple’s contact tracing techniques, which protect users’ privacy by encrypting all Bluetooth metadata and prohibiting the acquisition of location data.
De Montjoye expects that the findings will aid policymakers in developing better ways to protect consumers’ identities. According to him, data protection regulations allow for the exchange of anonymised data in order to encourage useful research. “However, in order for this to function, we must ensure that anonymization really protects people’s privacy.”