As summer-warmed waters eat away at the ice’s submerged edges, the Arctic Ocean’s sea ice will shrink to its smallest size this year in about a week.
According to scientists, this year’s sea ice levels will probably not break any records. A record low was reached by the ice cover in the Arctic in 2020, with 3.74 million square kilometers of ice covering that part of the land. At present, Arctic sea ice only covers about 4 million square kilometers, making this year’s extent the 10th lowest extent recorded since satellites began recording data in 1979. A surprising outcome considering sea ice levels were at a record low in early summer.
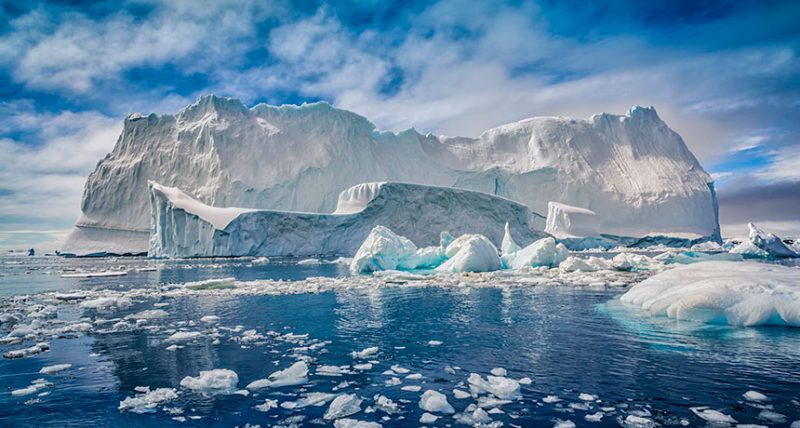
Due in part to the fact that the best current statistical- and physics-based models are only able to accurately predict sea ice extent a few weeks in advance, they are less accurate for long-term forecasts. Researchers report August 26 in Nature Communications that a new tool that uses artificial intelligence to forecast sea ice could boost their accuracy significantly.
According to BAS Artificial Intelligence Lab data scientist Tom Andersson, IceNet is 95 percent accurate in forecasting sea ice two months in advance – higher than the leading physics-based model SEAS5. The forecast produced by SEAS5 on a supercomputer takes about six hours, while IceNet on a laptop takes less than a minute. Andersson and his colleagues found that their algorithm can also predict unusual ice events four months in advance – unusual highs or lows.
To keep track of the impacts of climate change, sea ice must be tracked. However, IceNet’s advanced notices could also be beneficial more immediately. In this way, scientists could assess and prepare for the risks of Arctic fires and wildlife-human conflicts, and Indigenous communities could have the data they need to make economic and environmental decisions.
The Arctic sea ice extent has steadily declined since satellite measurements began in 1979 (SN: 9/25/19). The goal of improving sea ice forecasts has eluded scientists for decades. “Forecasting sea ice is really hard because sea ice interacts in complex ways with the atmosphere above and ocean below,” Andersson says.
A future prediction of sea ice changes is based on existing tools that convert the laws of physics into computer code. These models, however, struggle to predict the long-term state of sea ice in part because of uncertainties in the underlying physical systems.
To train IceNet to predict the state of sea ice in the future by analyzing past sea ice observations, Andersson and his colleagues used deep learning to combine observational data from 1979 to 2011 with climate simulations from 1850 to 2100.
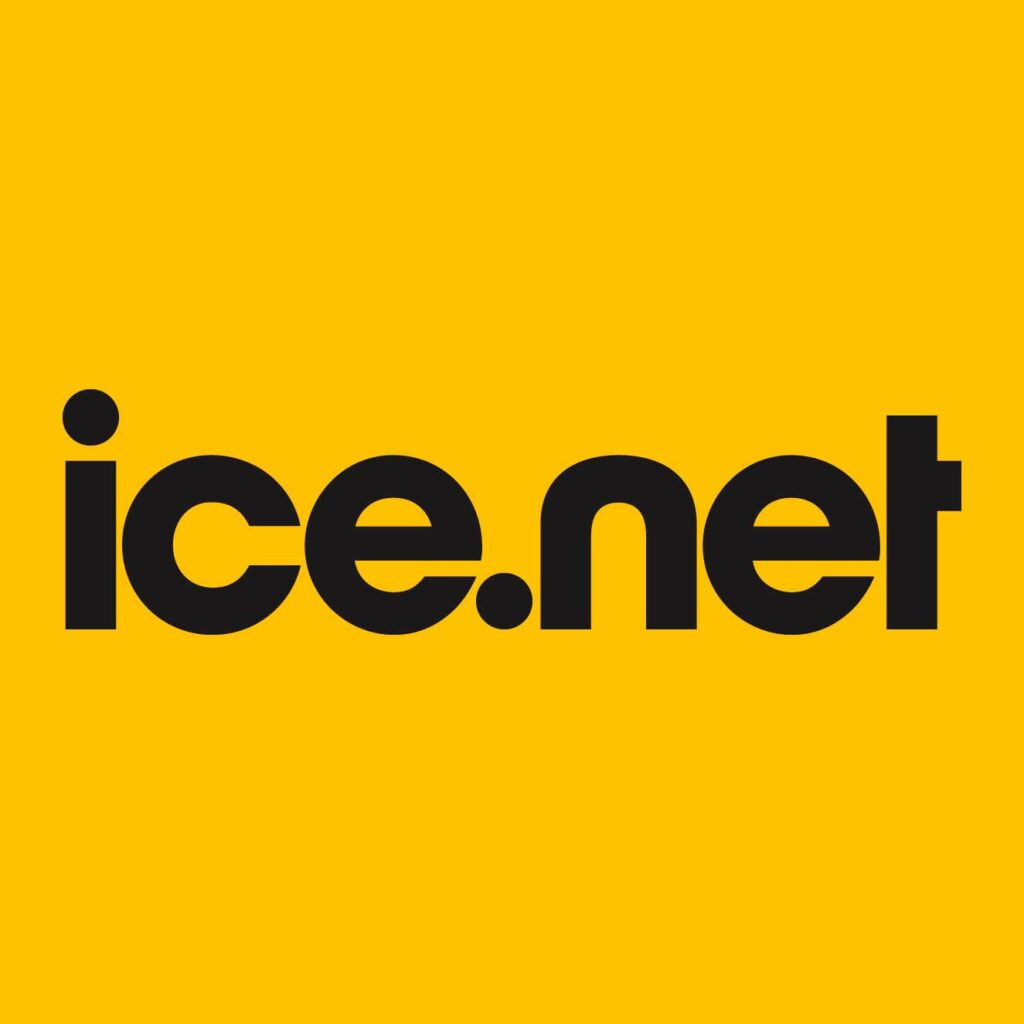
A comparison was made between IceNet’s outputs and the sea ice extent observed from 2012 to 2020, and the forecasts given by SEAS5, the widely used tool used by the European Centre for Medium-Range Weather Forecasts for making predictions. A further 360,000 square kilometers of ocean were properly classified as “ice” or “no ice” using IceNet as opposed to SEAS5.
Moreover, a drastic drop in summer sea ice extent in 2012 led to an all-time low extent in September. IceNet anticipated the dip without even having to run past past data. While SEAS5 had some inklings too, its projections beyond ten years were off by about a hundred thousand square kilometers.
“This is a significant step forward in sea ice forecasting, boosting our ability to produce accurate forecasts that were typically not thought possible and run them thousands of times faster,” says Andersson. It’s possible that IceNet has a better understanding of the physical processes determining the evolution of sea ice through training data than models based on physics.
“These machine learning techniques have only begun contributing to [forecasting] in the last couple years, and they’ve been doing amazingly well,” says Uma Bhatt, an atmospheric scientist at the University of Alaska Fairbanks Geophysical Institute who was not involved in the new study. Moreover, she is the leader of the Sea Ice Prediction Network, which brings together scientists from varied disciplines to improve forecasting.
According to Bhatt, good seasonal ice forecasts are important for assessing the risk of Arctic wildfires, which are strongly correlated with sea ice (SN: 6/23/20). “Knowing where the sea ice is going to be in the spring could potentially help you figure out where you’re likely to have fires — in Siberia, for example, as soon as the sea ice moves away from the shore, the land can warm up very quickly and help set the stage for a bad fire season.”
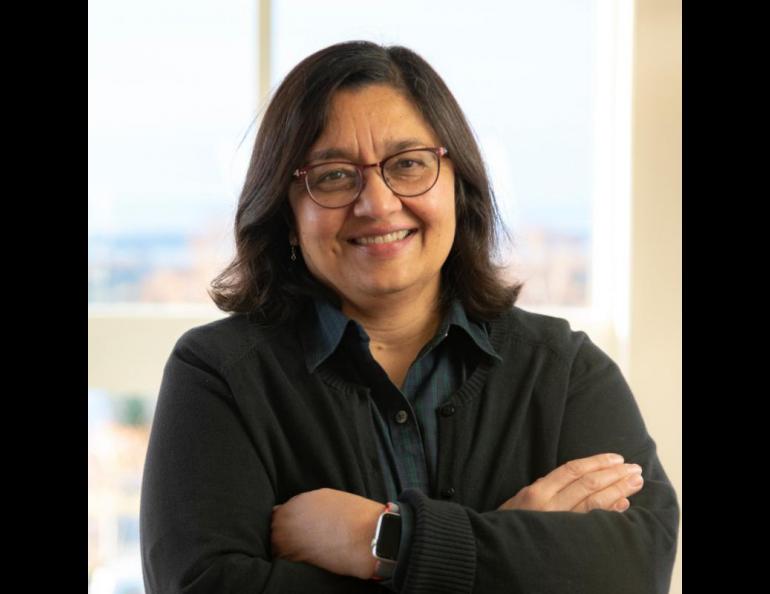
Any improvement in sea ice forecasting will also benefit northern and Indigenous communities in terms of economic, safety, and environmental planning. Several thousand walruses come on land during the sea ice disappearance to rest in the sun (SN: 10/2/14). In addition to triggering deadly stampedes, humans have also been found to cause high walrus mortality rates. Forecasts of seasonal ice loss can help biologists manage haul-out areas ahead of time by limiting human access to these locations.
Despite this, there are still limitations. 91 percent of the accurate predictions of September’s ice edge came from the system four months in advance. IceNet, like other forecasting systems, struggles to produce accurate long-range forecasts for late summer due, in part, to what scientists call the “spring predictability barrier.” Knowing the condition of the sea ice before the melt season begins is crucial for accurately forecasting end-of-summer conditions.
Another limit is “the fact that the weather is so variable,” says Mark Serreze, director of the National Snow and Ice Data Center in Boulder, Colo. Despite the likelihood of a new annual low of sea ice at the start of July, cool atmospheric temperatures ultimately slowed down ice loss. “We know that sea ice responds very strongly to summer weather patterns, but we can’t get good weather predictions. Weather predictability is about 10 days in advance.”